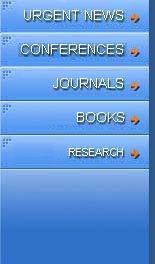 |
Main Page of the
Journal
Main Page of the WSEAS
Dec.2006, Jan. 2007,
Feb. 2007,
Mar. 2007, Apr.
2007,
May 2007,
Jun. 2007,
Jul. 2007,
Aug. 2007,
Sep. 2007
,
October 2007, Nov. 2007, Dec. 2007, 2008
WSEAS TRANSACTIONS
on
BIOLOGY and BIOMEDICINE
Issue 2, Volume 4, February 2007
Print
ISSN: 1109-9518
E-ISSN: 2224-2902 |
|
|
|
Title of the Paper: Effects of microarray noise on inference efficiency of a stochastic model of gene networks
DOWNLOAD FULL PDF
Authors: Daniel A. Charlebois, Andre S. Ribeiro, Antti Lehmussola,
Jason Lloyd-Price, Olli Yli-Harja, Stuart A. Kauffman
Abstract: The appearance of microarray technology led to the development of algorithms to infer the structure underlying the
dynamics of gene regulatory networks (GRNs) from gene expression data. Yet, this technique is currently highly noisy, leading
to the question of how inferable are GRNs from this data. To answer this question, we use realistic models of GRNs [1, 2, 3]
and artificially introduce realistic measurement noise [4] to the resulting time series. We analyze the inference efficiency
of IADGRN [5], an inference algorithm of structure and logic, from this data. Since microarrays are attained from multiple
identical cell measurements and the effects of averaging their expression levels is still poorly understood, we investigate
these effects on the mutual information between pairs of genes (pI) [6, 7] averaged over the time series of multiple identical
cells, which are assumed to be initially synchronized. We test noise level effects using two noise models (Rocke and hierarchical
error model, Hem). It is found that the Hem model more strongly disrupts correlations for low noise levels. We show that although
time series binarization causes information loss, it makes inference more robust to low levels of noise. Also, while indirect
interactions are not inferable even for low levels of noise, above a certain relatively small noise ratio even strongly correlated
genes lose any significant correlation for both noise models. This shows how inaccurate current inference algorithms are when inferring
from data with realistic characteristics.
Keywords: Gene Regulatory Networks, Delayed Stochastic Simulation Algorithm, Microarray, Inference.
Title of the Paper: Scene analysis using both a probabilistic evolutionary graph
and the evidence theory
DOWNLOAD FULL PDF
Authors: N. Ammour, A. Guessoum, D. Berkani.
Abstract: In this paper, we present a new scene analysis method based on the progress of a probabilistic
evolutionary graph. The strategy consists in making grow an evolutionary graph, which presents the scene
elements in an unsupervised segmented image. The graph evolution development is based on the computation
of the belonging probabilities to the existing classes of the last built region. The space composition matrix of
the areas in each class is then given. A space delimitation map of the regions is established by a new method of
contour localization and refinement. At last, the final segmented image is established by classification of the
pixels in the conflict region using the Dempster-Shafer evidence theory. The effectiveness of the method is
demonstrated on real images.
Keywords: Evolutionary graph, transition, segmentation, class, contour, region, Demspter-Shafer evidence
theory.
|
|
|